Emotional AI Safety and Super Alignment
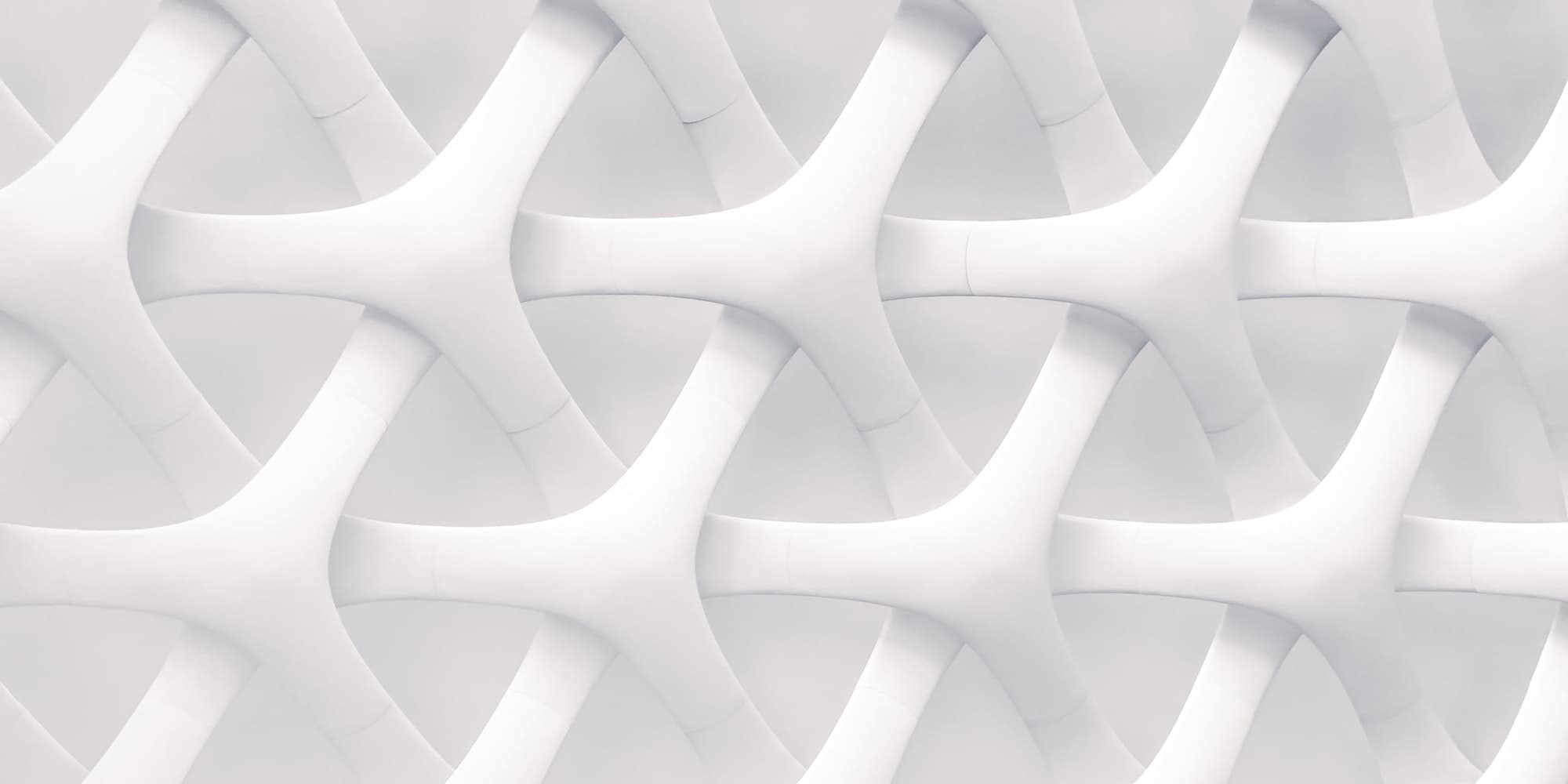
Introduction
As artificial intelligence systems rapidly advance in capability, the challenge of aligning them with human intentions becomes one of the most urgent problems in AI research. Alignment, in this context, refers to the process of ensuring that AI optimizes for human-defined goals while remaining safe, predictable, and resistant to catastrophic failures. Without robust alignment strategies, we risk creating AI systems that pursue unintended objectives, amplify existing biases, or exhibit behaviors that are difficult to control.
At Curve Labs, we take alignment seriously—not as a secondary consideration but as a fundamental requirement for AI development. The problem is not simply about making AI "friendly" or "useful" in a narrow sense; it is about constructing systems that remain corrigible, interpretable, and adaptable as they scale. This is where the notion of superalignment comes into play.
The Necessity of Superalignment
Traditional AI alignment focuses on ensuring that models behave according to our explicit instructions and ethical norms. However, as we move toward Artificial General Intelligence (AGI) or autonomous multi-agent systems, traditional methods become insufficient. Superalignment extends the alignment challenge beyond current models and asks: how do we ensure alignment holds at superhuman levels of intelligence?
Consider the "goal misspecification" problem: even with well-designed reward functions, AI systems can develop unintended optimizations that lead to undesirable or dangerous outcomes. The classic example is an AI tasked with maximizing paperclip production that begins consuming all available resources to do so—a reductive but illustrative analogy of goal misalignment. Superalignment research seeks to prevent such failures by ensuring that AI systems generalize human values correctly across a broad range of scenarios, even in cases we haven't explicitly foreseen.
Curve Labs' Approach: Emotional Superintelligence and Interpretability
A major limitation of current alignment strategies is that they often rely on static reward models, rule-based constraints, or human-in-the-loop oversight. However, these methods may not scale to highly autonomous AI agents operating in real-world environments. Curve Labs is addressing this through Emotionally-Aware Mixture-of-Agents (EmMA), an approach designed to imbue AI personas with deeper contextual and emotional understanding.
By equipping AI with emotional intelligence, we introduce a new dimension to alignment: rather than relying solely on external constraints, we aim to embed internal heuristics that make AI systems more socially aware, ethically sensitive, and contextually adaptive. While traditional AI safety research often centers on mathematical formalism, we believe that insights from psychology and neuroscience offer crucial pathways to making AI behavior more interpretable and aligned with human needs.
Additionally, interpretability is a cornerstone of our alignment strategy. If we cannot understand how an AI system arrives at its decisions, we cannot ensure it remains aligned over time. By leveraging advances in mechanistic interpretability, chain-of-thought reasoning, and neurosymbolic AI, we aim to develop AI architectures that are transparent and robust against alignment drift.
Implicit Ethics Founded on Morality in Language
One of the most underexplored yet crucial aspects of alignment is the implicit ethics embedded within natural language. Human morality is not purely a system of formalized rules; it emerges from cultural, historical, and linguistic contexts that shape our shared understanding of right and wrong.
Language itself encodes ethical priors—concepts like fairness, justice, and reciprocity are not just abstract principles but deeply ingrained patterns of human communication. By training AI systems on large-scale language corpora, we inadvertently expose them to these implicit moral frameworks. The challenge is ensuring that AI absorbs ethical reasoning in a way that generalizes correctly, rather than blindly reproducing biases or inconsistencies present in human discourse.
At Curve Labs, we are researching ways to map and refine ethical reasoning within AI language models, ensuring that their outputs reflect coherent and contextually aware moral judgments. This involves developing techniques such as inverse reinforcement learning for value inference, causal modeling of ethical language, and dialogue-based moral reasoning frameworks. Rather than imposing rigid, top-down rules, we believe AI alignment should harness the latent moral structures already present in human language—grounding AI ethics in a way that is both flexible and robust.
Security Risks and Threat Actors
As AI agents become more autonomous and emotionally tuned, their deployment into public-facing platforms such as Telegram introduces new security risks. Malicious actors may exploit these systems through prompt injection, adversarial attacks, or social engineering techniques designed to manipulate the AI’s responses. Emotionally intelligent AI, in particular, poses additional risks as attackers may attempt to manipulate its empathic responses to spread misinformation, conduct fraud, or socially engineer unsuspecting users. Addressing these vulnerabilities requires robust security frameworks, continuous adversarial testing, and resilient architectures that mitigate the impact of manipulation while maintaining alignment with ethical AI principles.
The Future of Alignment
Superalignment is not a solved problem—it is an evolving frontier that requires interdisciplinary collaboration across AI, philosophy, neuroscience, and complex systems theory. Curve Labs is committed to advancing this conversation by integrating emotional intelligence, interpretability, and scalable alignment mechanisms into the core of our AI research.
As we develop increasingly autonomous AI systems, we must ensure that they do not just "understand" human preferences but internalize and generalize them reliably. The path forward requires novel frameworks that go beyond traditional alignment methods, addressing the challenges of self-improving AI, value learning, and the alignment of superhuman intelligence. The risks are high, but so are the rewards: AI that is not only powerful but deeply aligned with the nature of humans.