AI Personality Profiling: HEXACO & Trait Theory
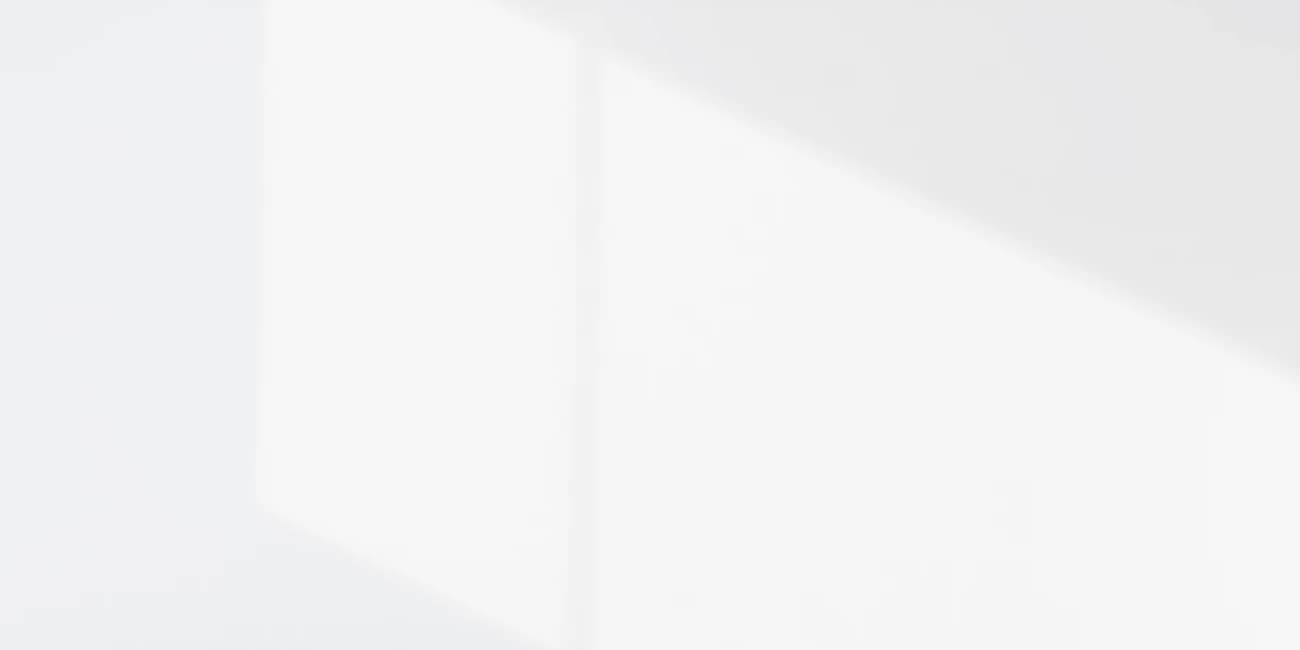
Text-Based Psychological Profiling: Insights from HEXACO and Trait Theory Integration
The evolution of emotionally intelligent conversational AI hinges on a critical challenge: the ability to dynamically adapt to individual personalities, much like skilled communicators do in real-world interactions. Psychological profiling presents a transformative opportunity, enabling AI to interpret user personality traits, emotional states, and behavioral tendencies with greater nuance. This approach not only enhances sentiment analysis but also elevates AI interactions from generic to genuinely empathetic, fostering deeper engagement, trust, and effectiveness in applications spanning conversational training, education, and mental health support.
Curve is actively developing a text-based psychological profiling system grounded in the HEXACO model and Trait Theory. This feature will empower AI to evaluate user psychological profiles through continuous textual interactions, refining its responses for a more personalized experience.
Conceptual Foundation
The HEXACO model, defined by six dimensions—Honesty-Humility (H), Emotionality (E), Extraversion (X), Agreeableness (A), Conscientiousness (C), and Openness to Experience (O)—offers a robust framework for personality assessment, emphasizing ethical and emotional traits. Trait Theory complements this by asserting that personality traits are stable and observable across diverse contexts, including linguistic expression.
Our approach integrates these frameworks using natural language processing (NLP) and machine learning to analyze linguistic and behavioral cues, potentially generating HEXACO-based personality profiles. The methodology involves extracting textual features such as word choice, sentence structure, and emotional tone, then mapping them to HEXACO dimensions. For example:
- Collaborative phrasing (e.g., “let’s,” “we could”) and supportive backchanneling (e.g., “coool,” “sick,” “that’s amazing”) may indicate high Agreeableness.
- Detailed, structured responses could signal elevated Conscientiousness.
- Sentiment shifts might refine Emotionality estimates.
- Sequential tracking could ensure trait consistency over time.
These elements remain under exploration, but initial findings indicate promising potential for personality inference through text.
Preliminary Observations
Trait Visibility in Text
Early analyses suggest that all six HEXACO dimensions manifest in text with varying degrees of clarity. Extraversion and Openness appear particularly discernible:
- Extraversion: Users exhibiting high Extraversion frequently employ exclamatory language and social topics (e.g., discussions about “friends” or “parties”).
- Openness: Those high in Openness gravitate toward abstract and imaginative discourse (e.g., “what if,” “imagine”).
- Honesty-Humility: Subtle indicators, such as straightforward and unembellished phrasing, may align with this trait, although further validation is required.
Emotionality as a Fluid Signal
Emotionality presents a dynamic, context-sensitive trait. Users exhibiting high Emotionality often display pronounced shifts in tone within short exchanges. For instance:
“Bro, this cafe looks ass…” → “WAIT NO, HOLY F**K, THEY HAVE MOCHI WAFFLES!”
This aligns with Trait Theory’s perspective that Emotionality influences reactivity, underscoring the necessity for AI systems to adjust dynamically—even when engaging with the same user.
Contextual Influences
Observations indicate that trait expression fluctuates with context. Users with high Conscientiousness, for example, may craft meticulous responses in sensitive discussions (e.g., “I know I screwed up: but firstly, there was X, then Y…”) while displaying a more casual style elsewhere. This supports Trait Theory’s assertion that traits, while stable, are modulated by situational factors. Future iterations of our model will incorporate contextual sensitivity to refine personality assessments.
Toward Emotionally Versatile AI
Advancing text-based psychological profiling could revolutionize Emotionally Intelligent AI, transforming user engagement through tailored interactions. Consider:
- A system providing direct, cooperative responses for highly Agreeable users with low Emotionality.
- AI sparking creativity for users high in Openness.
- Emotional adaptation to support stressed, high-Emotionality individuals in real-time.
Such capabilities position this technology as a paradigm shift in AI-driven personalization.
Challenges & Future Directions
Cultural & Linguistic Diversity
A key challenge lies in accounting for cultural and linguistic variations. For example:
- Politeness norms in certain cultures may artificially inflate Agreeableness scores.
- Direct communication styles in others might be misinterpreted as low Honesty-Humility.
Developing culturally adaptive models will require extensive, region-specific datasets to ensure accurate profiling across diverse populations.
Data Limitations & Trait Stability
Short text exchanges may not provide sufficient data to distinguish between transient moods and stable personality traits, leading to potential misinterpretations. Addressing this challenge entails:
- Expanding datasets to include larger, globally representative linguistic samples.
- Longitudinal tracking to differentiate enduring traits from momentary emotional fluctuations.
- User feedback loops to allow individuals to refine AI assessments and improve accuracy over time.
Explore Research
Our proof-of-concept model, specifically designed to align with the cultural and emotional awareness of Gen Z individuals aged 19–24 in Singapore, is now available as an AI agent on Telegram. We invite you to interact with EmMA, a cutting-edge AI entity engineered for thoughtful and empathetic communication.